A machine learning toolkit for automated processing of multiplexed immunofluorescence images
Poster
November 1, 2023
Multiplexed immunofluorescence imaging is a powerful spatial biology tool that can produce rich marker expression data at single-cell resolution and wholeslide scales. High parameter images have been particularly useful for characterizing the composition and arrangement of cellular microenvironments and cell interactions in native tissue contexts. These spatial features have been shown to have predictive or prognostic value in clinical tasks, including riskbased cohort stratification, biomarker discovery, therapy response, and survival prediction across different cancers (1–4). Analyzing multiplexed immunofluorescence images can be fraught with both technical challenges, including tissue quality, stain quality, and artifact removal; and computational challenges, such as the processing, curation, and analysis of extremely large
raw image files. Excessive time spent manually annotating images, checking image and stain quality, aligning multiple images for multimodal analyses, and creating regions of interest to include or exclude in downstream analysis severely limits the throughput and utility of this technology. To address these challenges, we have developed and tested a suite of deep learning models and image processing utilities in a flexible, performant, and easy-to-use Python toolkit. Additionally this toolkit seamlessly plugs in with our data abstraction package emObject (5), which enables easy interaction with data in the Enable Medicine ecosystem. These tools allow analysts to prepare multiplex immunofluorescence images for accurate analysis at scale in an automated
fashion.
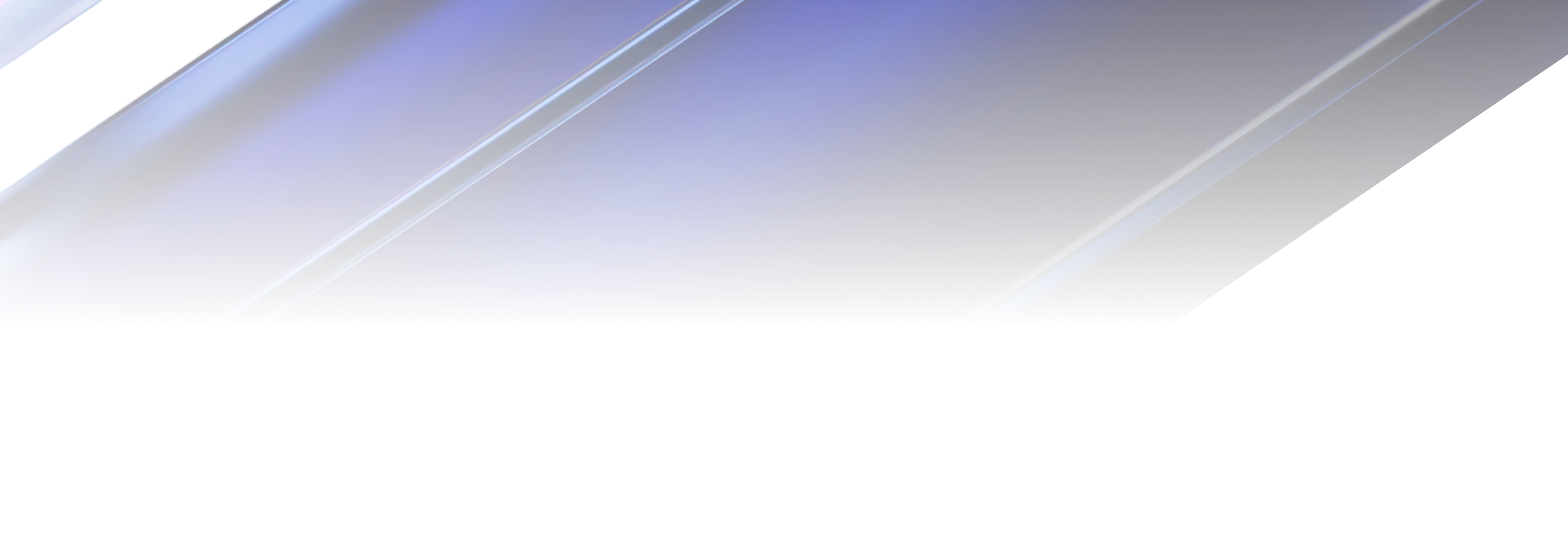