Why Spatial Biology


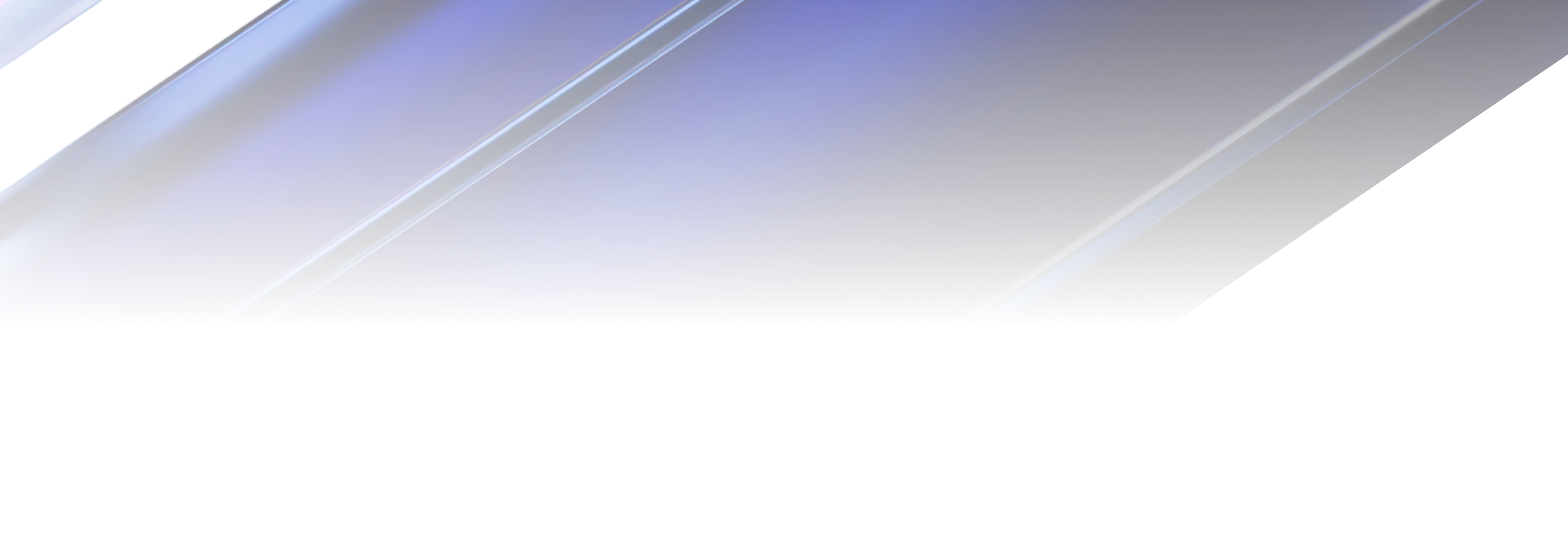
Spatial biology broadly refers to analysis methods which preserve information about the spatial organization of the biological specimen or system. By this definition, spatial biology is not new — one could argue we started studying living things by observing their spatial characteristics: anatomical features, movements, geographic/ecological locations, and others.
What is new is the resolution at which we can acquire spatial biology data (down to the single-cell and sub-cellular level, or even to the molecular and atomic level), the types of information we can extract (genomic, transcriptomic, and proteomic data), and the amount of data that we can store. Together, these three advances allow us to generate and analyze large spatial biology datasets and see how molecular- and cellular-scale features give rise to important biological outcomes at the organism scale. This information might, for example, provide insight into how tumors are organized to resist immune surveillance or the mechanism of action for a therapeutic agent.
But, how can we make sense of spatial datasets to gain insights from them, and what are its limitations?
What information can be gained from spatial analysis?
To state the obvious, spatial information allows us to better understand the organization of a sample or system.
A common analogy is that spatial data makes the difference between having a map of where people are in a city, versus having only a list of people in the city. With a list, we could determine that there is a higher number of people in one city compared to another, but we would have difficulty identifying which individuals are likely to encounter one another and variations in population density within the city. In some cases, the spatial information might affect decisions regarding resource allocation and districting. Clearly, spatial information is useful to have.
In the cell-to-tissue scale, the preservation of tissue structure allows us to map out the spatial relationships between entities, such as cells and/or cellular neighborhoods. This information improves our mechanistic understanding of the tissue. For example, if a tumor did not respond to a therapeutic, we might be able to determine if the effector T cells, which are required to kill cancer cells, penetrated the tumor region. Alternatively, we might find that there was a high presence of T regulatory cells that are known to suppress the immune function of effector T cells within the tumor. The information on the location of the T cell subtypes gives insight into why a tumor may or may not have responded to a therapeutic.
This stands in contrast to conventional non-spatial assays, such as western blots and flow cytometry, which destroy the larger scale structures of the tissue sample during processing. This makes it difficult to infer the organization or existence of multicellular structures and interactions. To go back to our tumor example, using a non-spatial method like flow cytometry, we would only be able to detect the presence, absence, and number of each T cell subtype.
For cell-to-tissue scale spatial biology, most spatial datasets are (or can be represented by) images, which inherently cover a range of scales – that is, features and objects within spatial datasets can be big or small relative to the whole image. For example, in a hematoxylin and eosin-stained (H&E) histology slide, we can identify nuclei and visualize protein presence, individual cells, multi-cellular structures such as glands or blood vessels, and neighborhoods such as the papillary dermal layer or epidermal layer in a skin sample. Thus, we can often infer many layers of spatial information even with very limited stains.
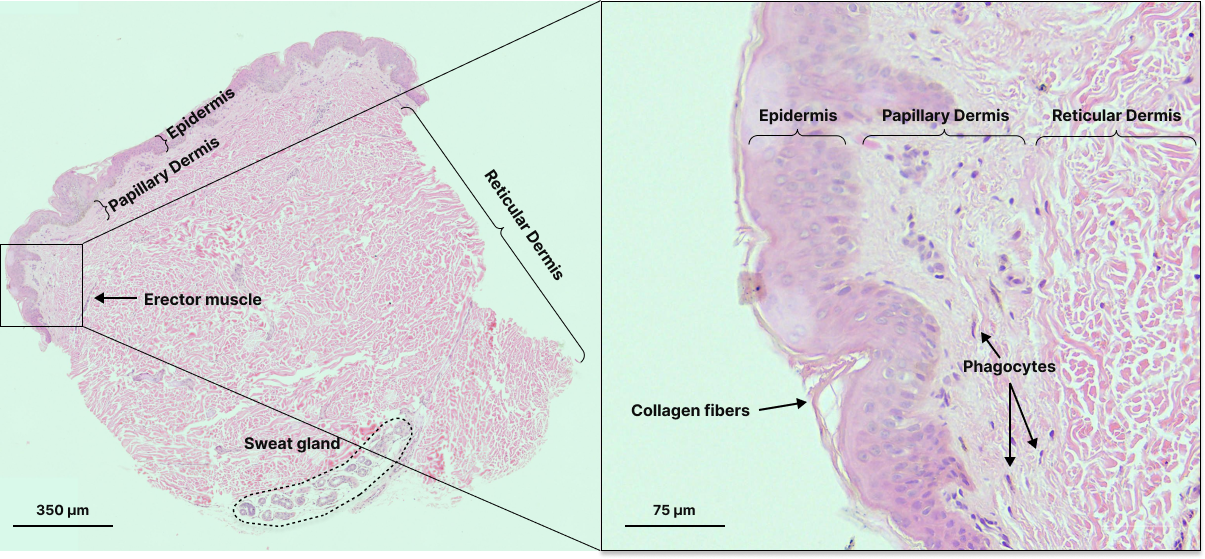
Hematoxylin and eosin stained skin sample with labels for features at various scales.
Scale is especially important to consider when inferring interactions between entities because of the physical limitations on signal transduction, and distance between objects and/or features is a uniquely spatial metric. Distances define the physical relationship between entities, dictating what modes of communication and what response timescales are possible. For example, cell-cell communication through paracrine signaling is diffusion-limited, so the distance measurements between cells can be used to identify which cells are able to communicate to each other. Distance measurements can also be used to quantify the degree of immune infiltration into a tumor or the distributions of distances between various cell types, which may give us information about cell migration or proliferation, and organization into spatial “neighborhoods”, which affect tissue structure and function.
Spatial data also naturally lends itself to morphology analysis. Nothing will convey morphological information as directly as an image. Furthermore, the morphological features of a tissue are not confined to cellular structures, and imaging tissue is one of the few ways that acellular structures can be detected and analyzed. Feature morphology is used heavily by pathologists to classify immune cell types and disease progression (e.g. Gleason score for prostate cancer), but automated pipelines for analyzing morphological features remains an active area of research and is likely underutilized at present.
Considerations to using spatial data
Of course, as with any technology, there are caveats and limitations to interpreting and analyzing spatial data.
One major limitation is file size — spatial datasets are extremely information rich, but the tradeoff is that they tend to be huge. Labs that generate these datasets may be managing hundreds of TBs of images and related metadata. Managing and operating on these large files often requires specialized hardware/computing systems and analysis methods.
Datasets can be reduced to more manageable sizes when we abstract away from the raw data files (i.e., images) using object segmentation, where pixels in the image are either automatically or manually grouped into features/objects. However, the level of abstraction must be carefully chosen since it defines how the image can be analyzed and interpreted. If we are interested in cell-level interaction, we might consider the natural choice for a segmentation unit to be a single cell, as it would be the smallest unit of object of interest. Individual cells could then be assigned properties such as x-y coordinates, biomarker expression levels, and morphology. However, by only segmenting by cells, we would be underutilizing the image data because we would miss any acellular signal. General segmentation models (e.g., Segment Anything), which detect objects or features at multiple scales could be developed to work around this, but the resulting abstracted datasets will still be quite large.
Furthermore, though analysis of these images will likely rely on automated segmentation due to the number of features and cells that need to be detected, no true general model exists for biological specimens. Current segmentation tools exist for single-cell segmentation as well as specific tissues and diseases rather than across scales and features. Large datasets with expert manual annotations of tissue structures are needed to develop truly robust models for general segmentation.
Even analyzing the abstracted datasets can take significant computational resources. These resources may be difficult for individual labs to access and/or maintain. Cloud-based computation can be a good workaround, but it can also be expensive.
The accuracy of segmentation in assigning pixels to a feature or object is also important to consider, since this assignment is how signal is attributed to objects such as single cells. Segmentation accuracy relies on the quality of the underlying data, which in turn is limited by the instruments of data acquisition. For cell-to-tissue scale spatial biology, microscopes and cameras are the typical acquisition devices. The instrument limits on pixel size and resolution, signal-to-noise ratio, depth of focus, etc. dictate the achievable image quality. Because of these limits, it is sometimes not possible to definitively attribute signals to the correct cell, especially in cases where cells are in close enough proximity to have overlapping pixels.
Cells in close proximity may have overlapping signal which cannot be accurately separated through segmentation algorithms. DAPI (blue) and PanCK (green) are displayed in this example taken from a non-small cell lung cancer sample.
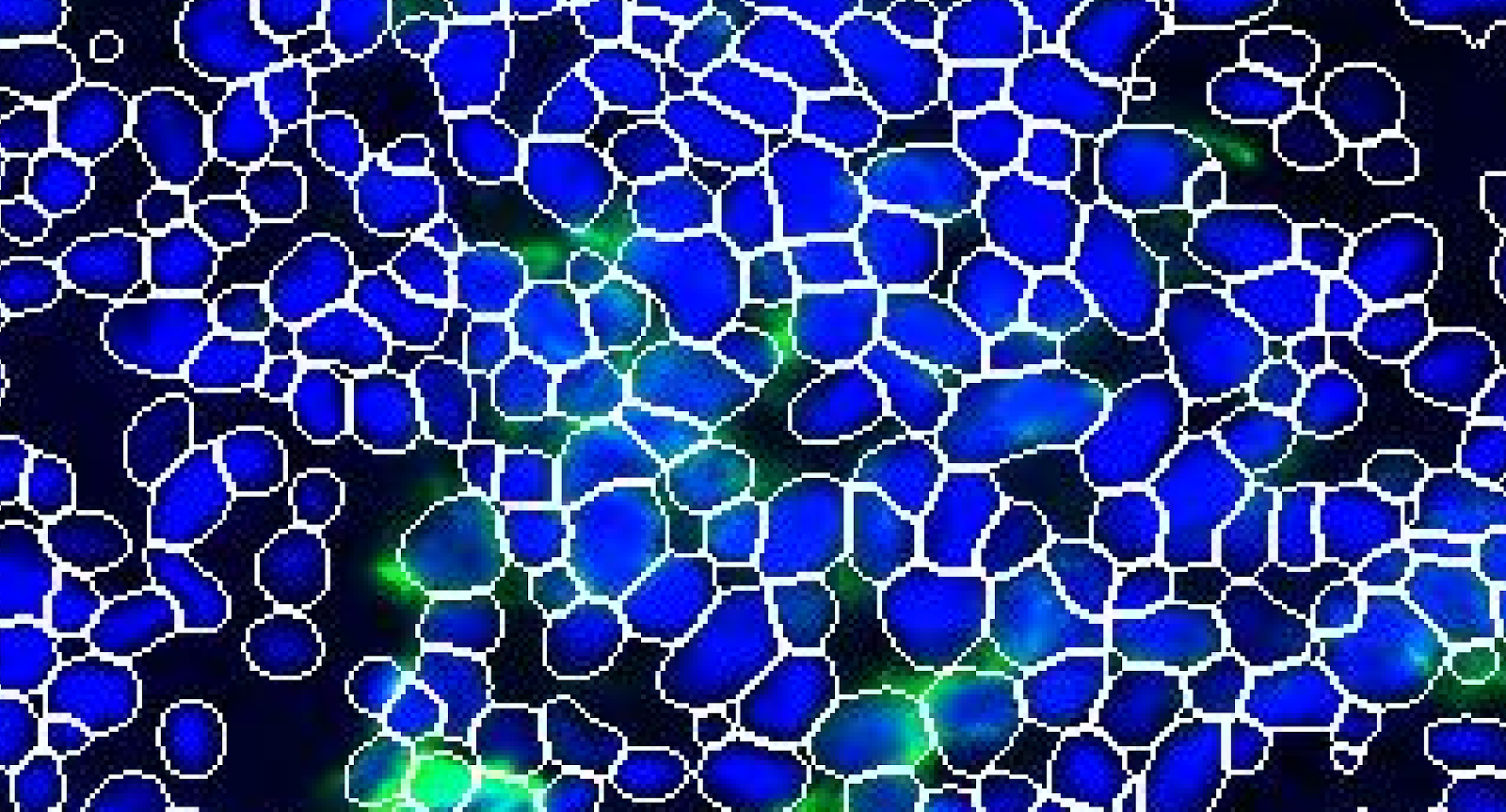
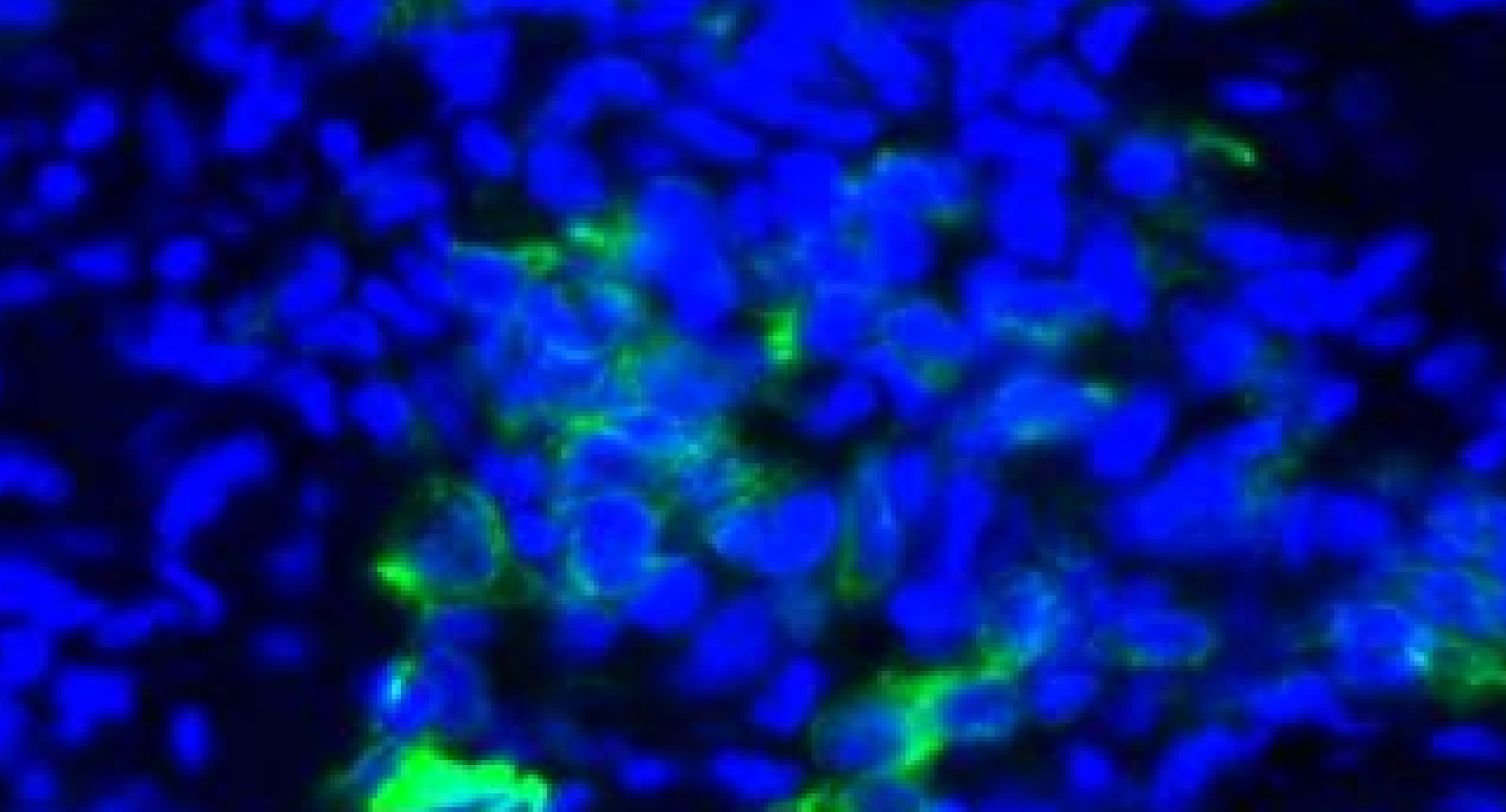
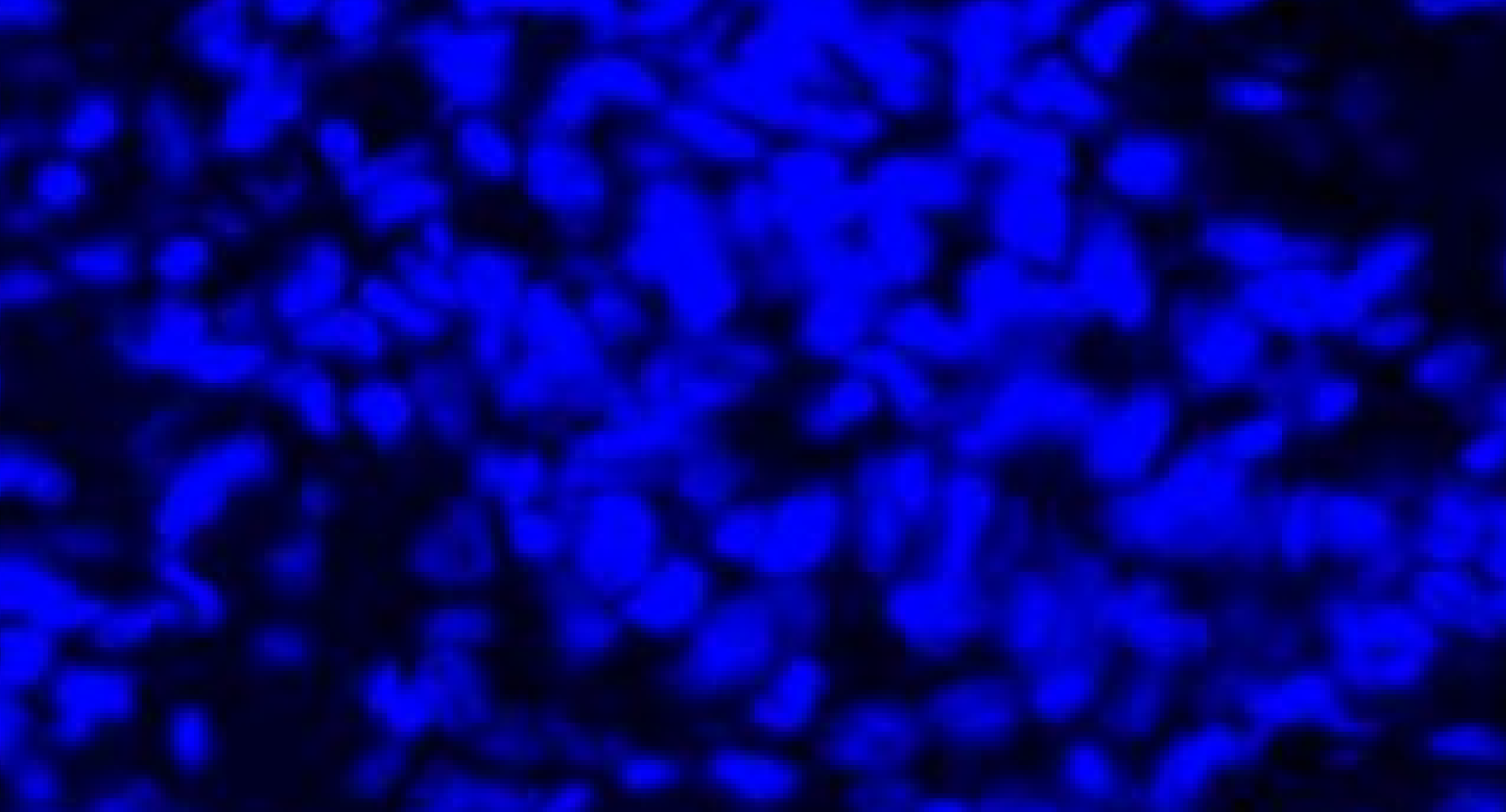
Importantly, this means that there are caveats to employing single-cell analysis approaches that were originally designed for scRNAseq or flow cytometry data, where individual cells are separated out and thus signals are well partitioned. While these approaches are frequently used because they are well-established and understood, there is an exciting opportunity to develop new methods (e.g., Pixie) that leverage spatial information for analysis.
Conclusion
Spatial biology is extremely rich and powerful, providing a lot of information about a wide range of features at different scales.
To take full advantage of the richness of spatial datasets, we will not only need to improve data storage and computation options, but also generate training data from a large number of well-annotated datasets that identify features over many scales. Furthermore, developing methods that take advantage of the spatial information available can also greatly improve interpretation of these datasets.
Spatial biology cannot yet replace other non-spatial methods wholesale, nor will it likely ever do so. Rather, it can provide unique insights into interactions and structures at multiple scales that can greatly augment our understanding of biology when used in conjunction with other methods. Already, spatial proteomic and transcriptomic datasets provide bridges between functional single-cell measurements and histopathology images. The combined findings of all of these assays enhance not only our understanding of structure and function relationships in tissue, but also our ability to glean information from each individual assay, making our suite of tools ever more powerful.

