Enable Medicine Develops a Deep Learning Model that Establishes a New Standard for Predicting Cancer Treatment Outcomes
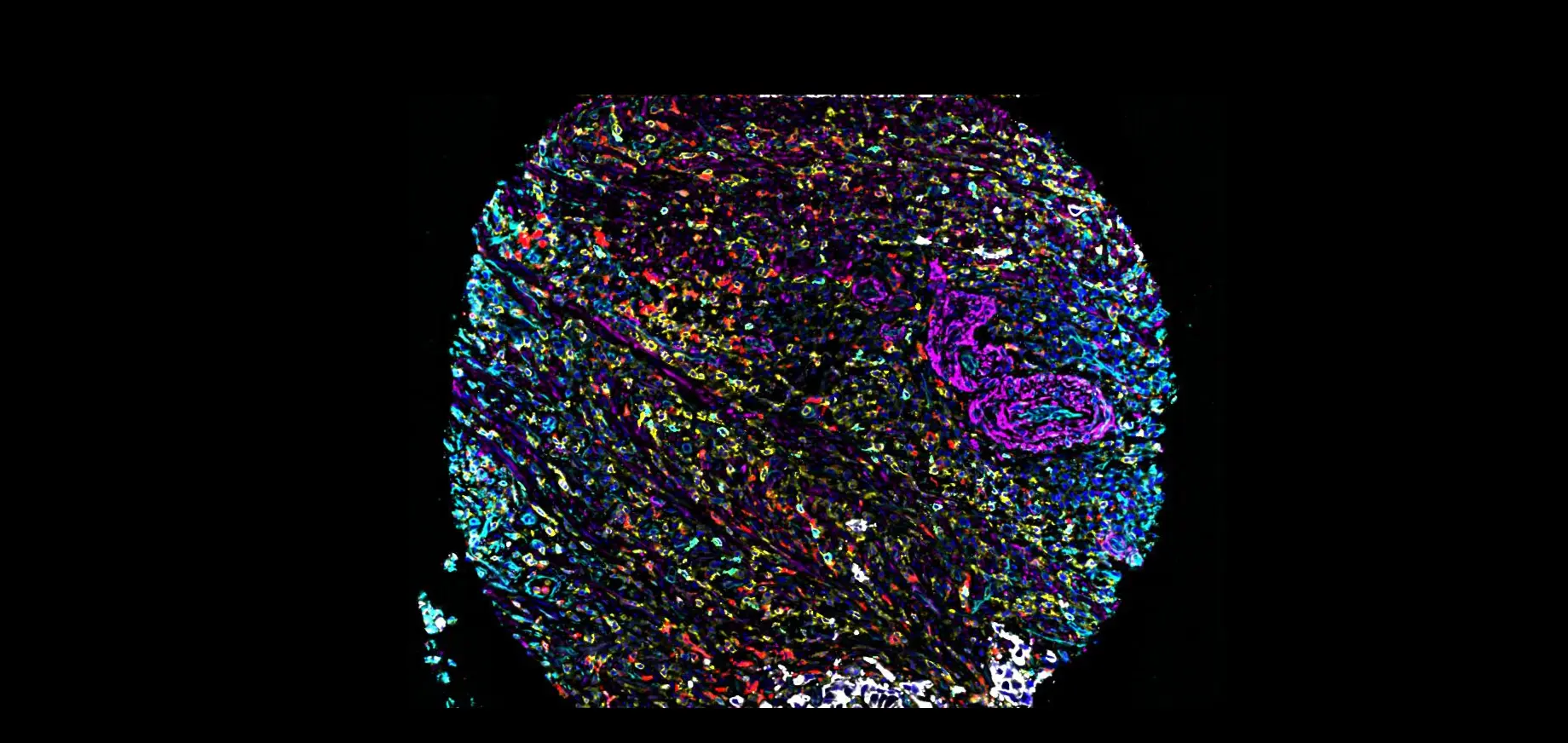
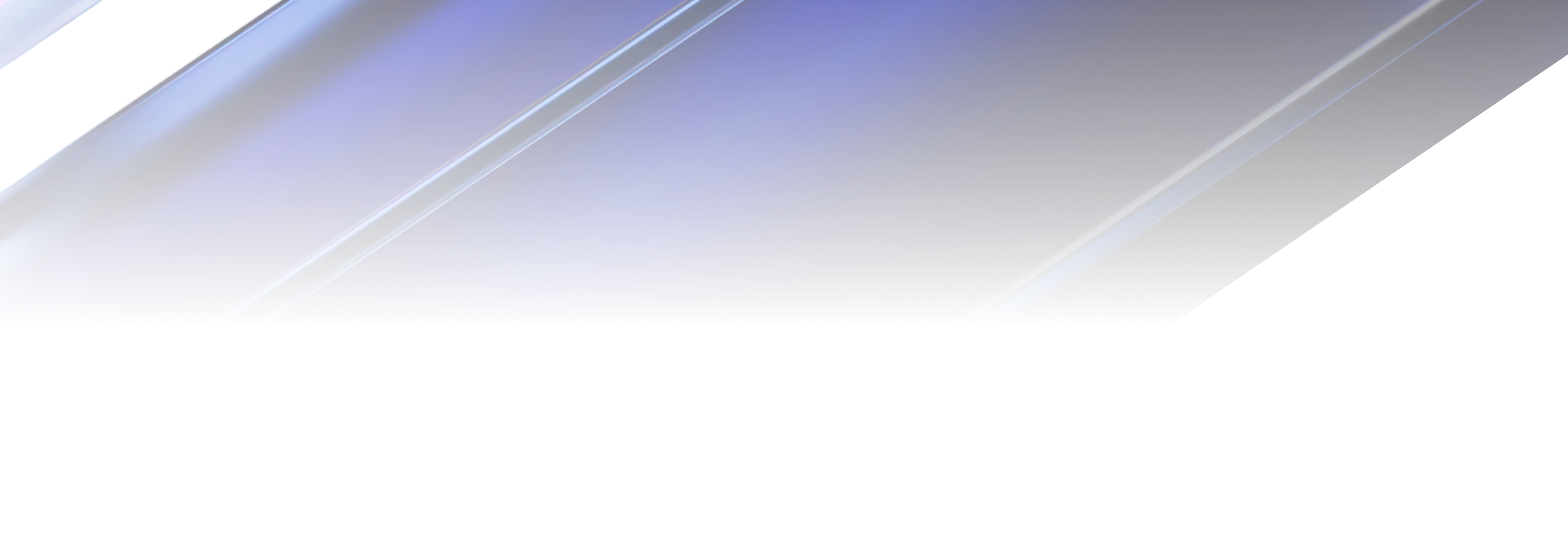
MENLO PARK, Calif., Nov. 10, 2022 (PRNewswire) -- Enable Medicine, the platform for indexing and searching biological data, today unveiled a new tool that identifies spatial-cellular signatures associated with cancer outcomes. Published in Nature Biomedical Engineering, scientists from Enable Medicine and the Stanford AI Lab detail how spatial cellular graphical modeling (SPACE-GM) substantially outperforms other state-of-the-art approaches at predicting cancer treatment outcomes. Quantitatively, the model achieves an area under the curve (ROC-AUC) above 0.85 for outcome classification across hundreds of patient tissue samples. The authors demonstrate that SPACE-GM generalizes predictions to unseen cancer types as well. The model is also interpretable, identifying the cellular configurations that contribute most to the predictions. This has broader implications for drug discovery and differential diagnosis.
Recently, in-situ biological characterization assays, including spatial transcriptomics and multiplexed immunofluorescence imaging, have enabled the rich characterization of tumor microenvironments from patient tissue samples. However, extracting biologically meaningful spatial signatures from these rich data remains an open challenge. Efforts to connect the spatial arrangement of cells in tissues with clinical results have met with limited success. SPACE-GM models tissues as graphs, where the nodes are cells and the edges encode relative physical proximity. In contrast to other similar methods, which are based largely on unsupervised characterization of cells and cellular neighborhoods, SPACE-GM was trained to directly predict patient prognosis or treatment response from cellular "sub-graphs." This allowed researchers to identify sub-graphs that were consistently associated with positive or negative outcomes. In subsequent interpretive analyses, the team identified a spatial signature characterized by mixed tumor subtypes that appeared more often in patients with worse outcomes. SPACE-GM predictions were correlated with the spatial compartmentalization of these subtypes, suggesting that the mixing of these subtypes was a negative indicator for prognosis. In another example, the spatial dispersal of granulocytes within tumor cells was associated with poor patient prognosis.
The development of the deep learning model leveraged Enable Medicine's rapidly expanding multiplexed imaging database and analytical expertise. The complete study was trained and evaluated with over 650 patient tissue samples collected independently from 3 institutions (Stanford University, Dana Farber Cancer Institute, and University of Pittsburgh Medical Center) including head-and-neck and colorectal cancer samples, demonstrating cross-tissue generality of the model. Each sample was stained with over 40 biomarkers. The data were processed using analysis pipelines custom built by Enable scientists and engineers.
The results demonstrate the power of Enable's ecosystem approach, where new models can be rapidly deployed on data collected and integrated at scale. The model itself also furthers Enable Medicine's company vision for biological search, where complex questions about human disease can be asked and answered for the first time.
"The cellular sub-graphs revealed by SPACE-GM are a huge step toward defining representations of biological data that can be queried and compared across tissues and diseases," said Alexandro Trevino, co-author and Machine Learning Team Manager at Enable Medicine. "Imagine an atlas of sub-graphs, which could allow the retrieval of similar cellular structures for a new patient sample. Such a resource could help make personalized treatment choices or guide hypothesis generation for novel drug discovery."
About Enable Medicine
Enable Medicine is the platform for indexing and searching biological data. The Enable platform unlocks the ability to search biology, where complex questions about human disease can be asked and answered for the first time. Our network of partners at leading academic institutions and pharmaceutical companies around the world use the platform to guide novel drug target discovery, identify biomarkers of therapeutic response, and develop optimal treatment strategies.
To read the paper in full, please find it here. Additional research can be found here.
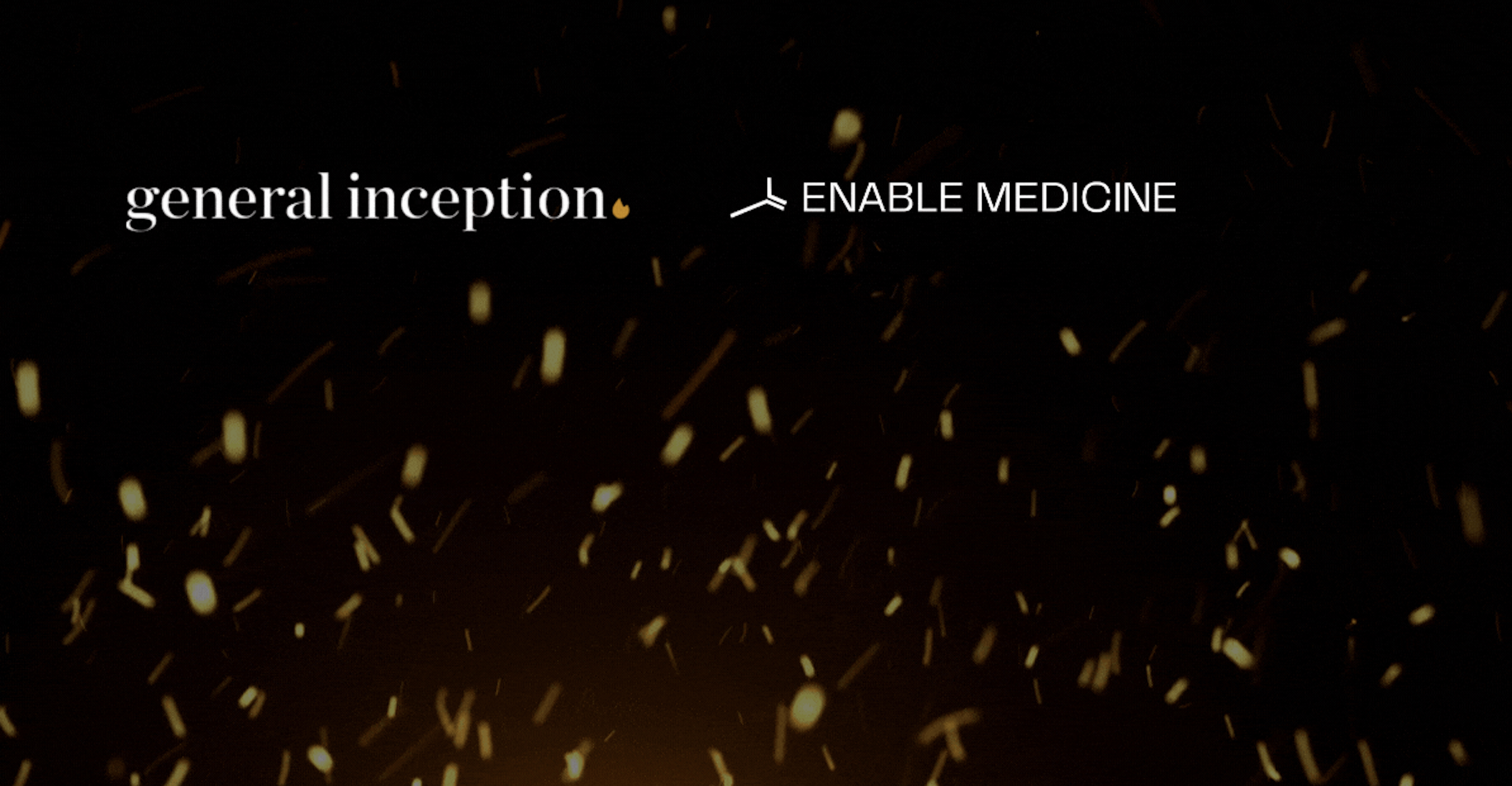
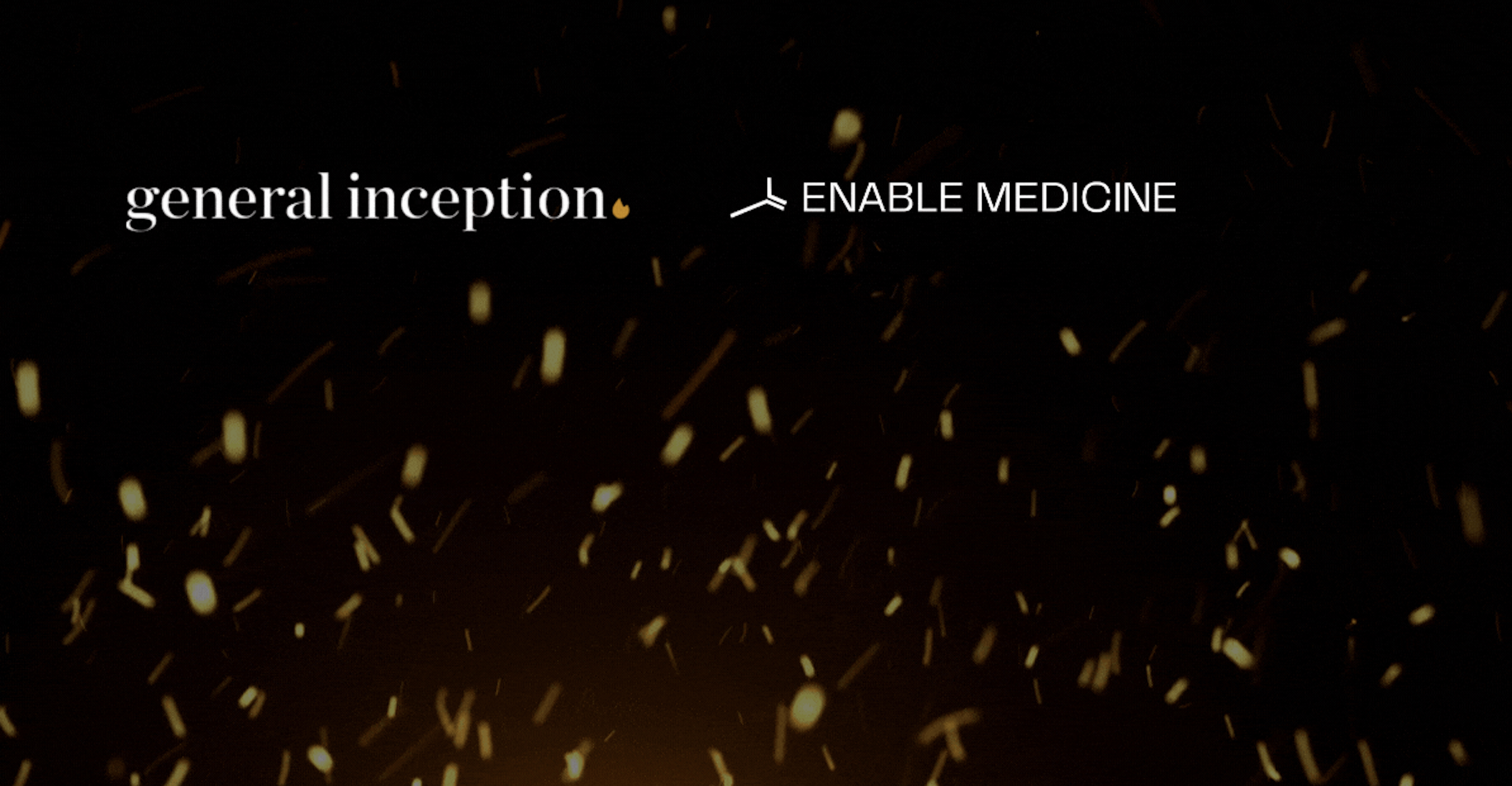


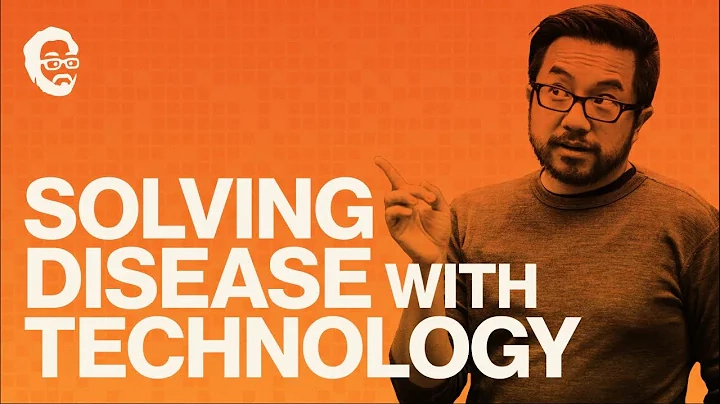
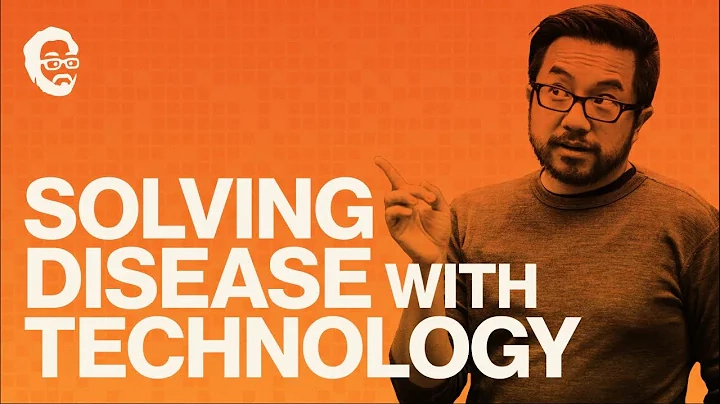




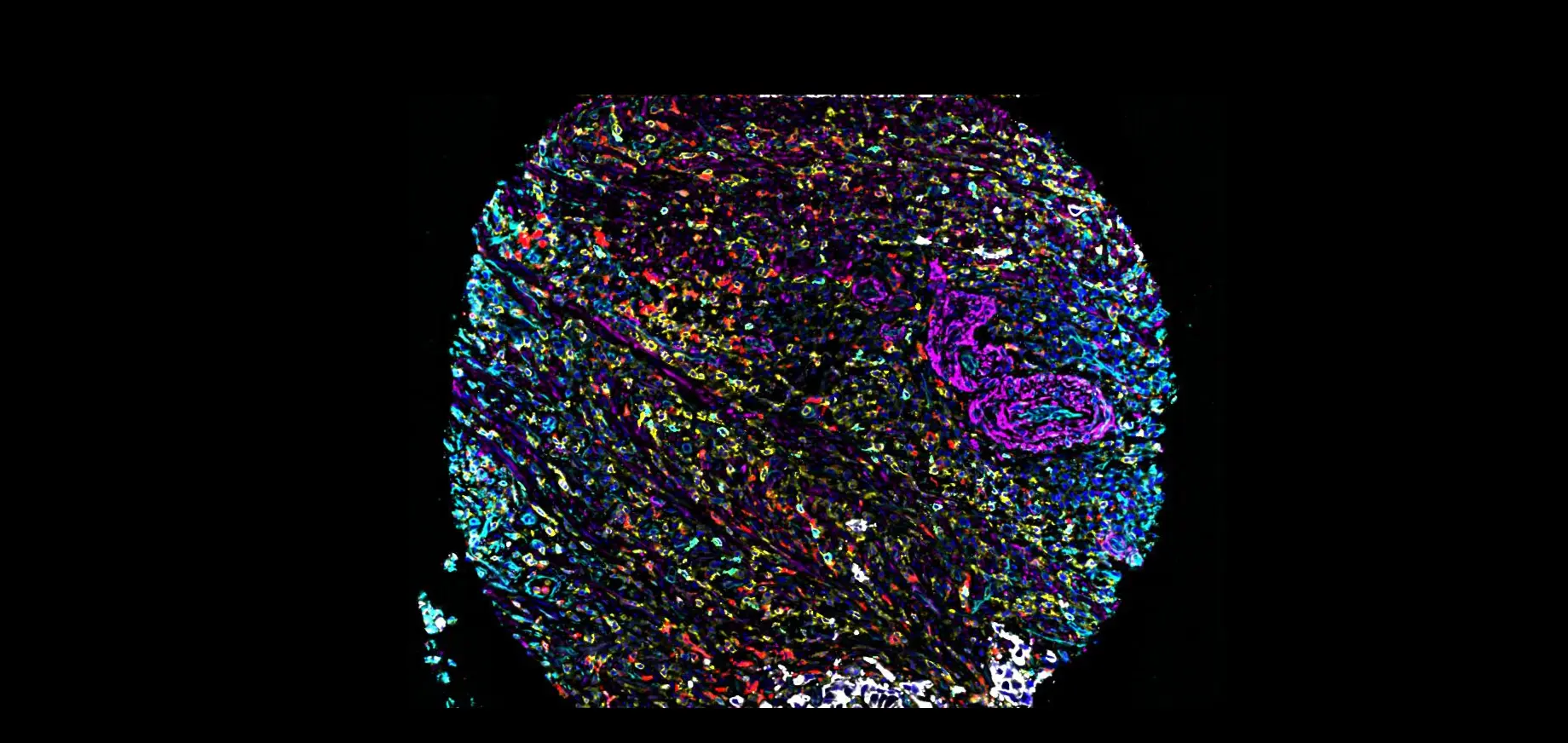
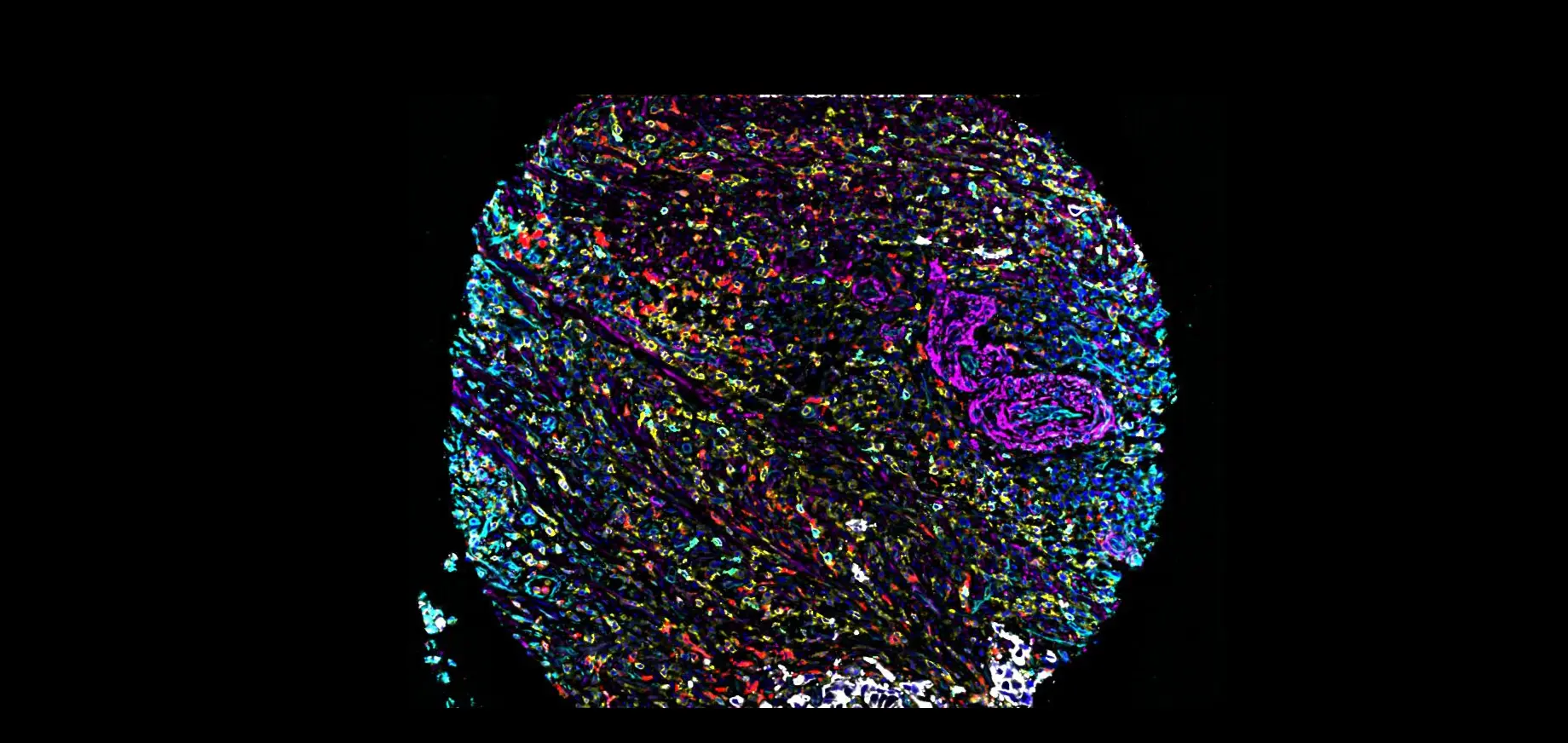
